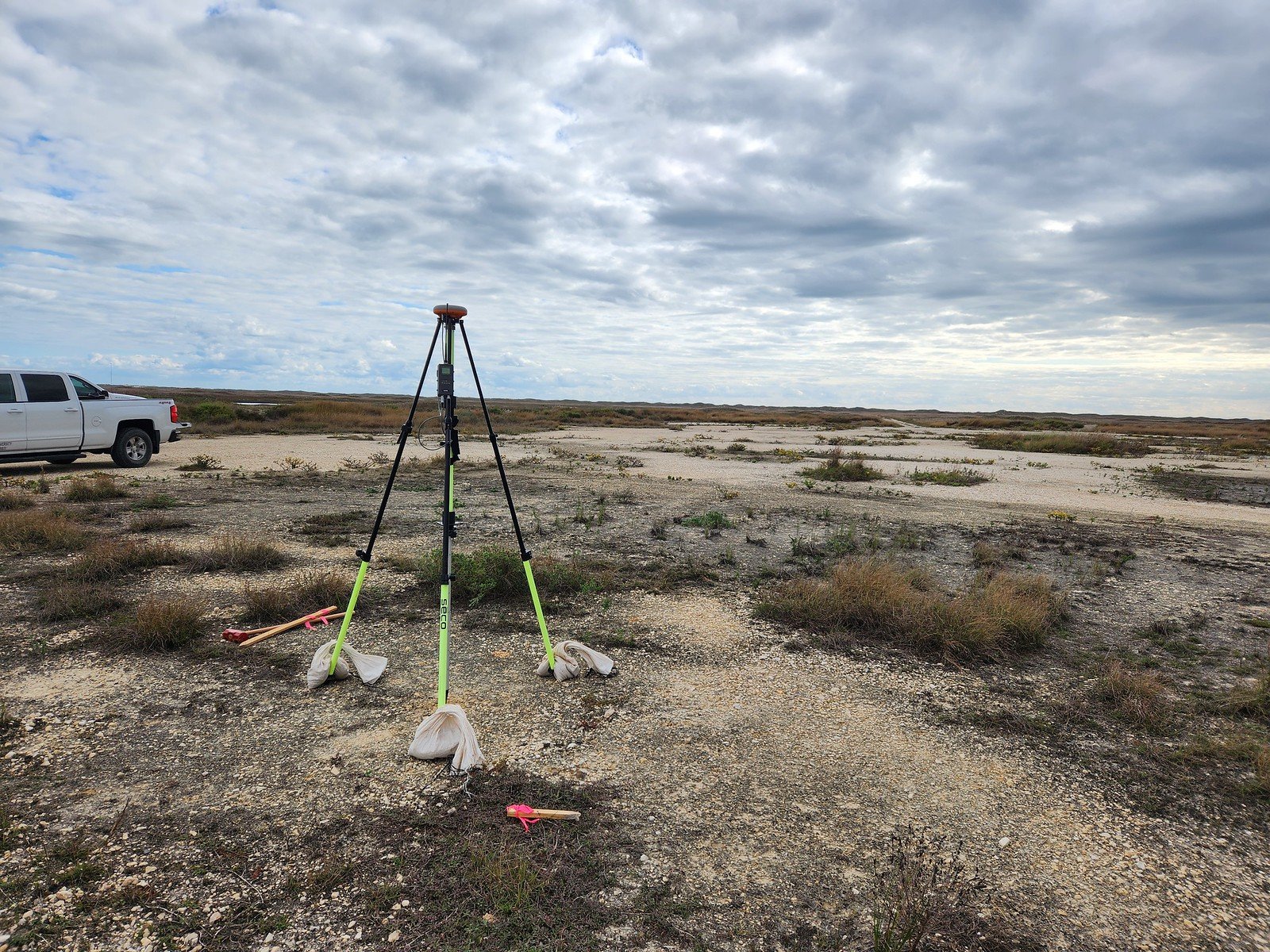
Projects
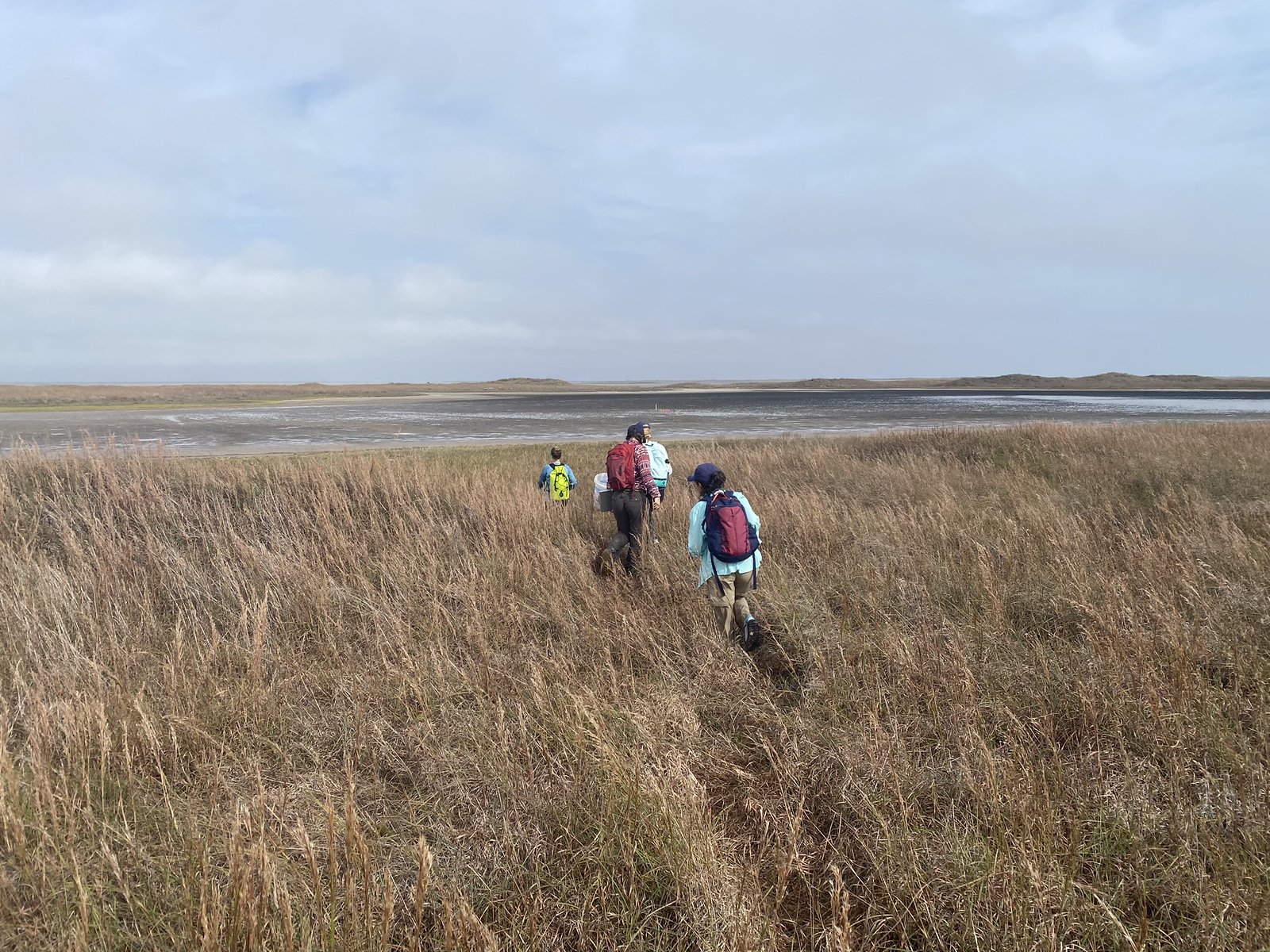
Coastal Inundation Project
Coastal inundations can have a major impact on beach management and safety. The frequency of flooding is increasing exponentially with relative sea level rise. This creates a need for more accurate models that can predict potential coastal inundation and alert beachgoers and officials of related hazards. The team is working on the creation of an AI inundation model capable to predict where the water will reach on the beach. This will be an invaluable addition to models that predict water levels at tide gauges but without including the water runup on the beach. Models predicting inundations on an hourly basis will help counties and cities better manage their beaches.
The Coastal Inundation Project has:
- Created a dataset of over 3,000 drone based coastal images from 12 locations in Florida and Texas with labeled shoreline wet/dry line including elevations and Deep Learning predictions.
- Undergraduate students developed water level predictions visualizations for stakeholders and scientists.
- A set of cameras including a stereo camera are being installed on a local pier to provide point cloud time series
- A peer reviewed conference paper (Vicens-Miquel et al.) “’Deep Learning Automatic Detection of the Wet/Dry Shoreline at Fish Pass, Texas” was accepted for IGARSS 2022 proceedings about to be followed by journal paper submission.
(reported by the National Science Foundation, 2022)
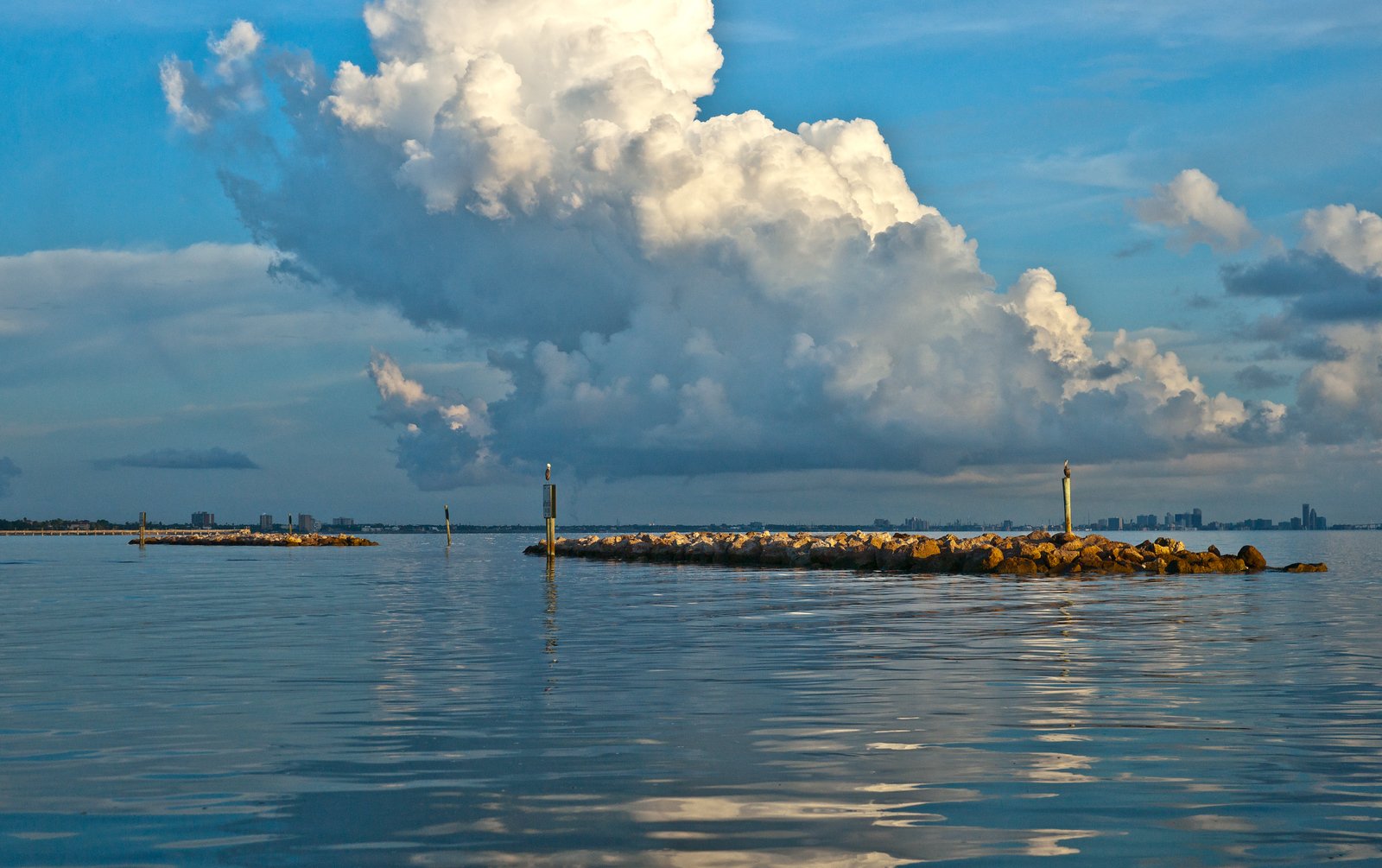
Sea Turtle Conservation
The Laguna Madre is home to many fish and sea turtle species, including juvenile green sea turtles, who take advantage of this rich and unique ecosystem. When strong cold fronts reach the South Texas coast, water temperature can decrease rapidly in the shallow waters of the Laguna Madre and other Texas Bays. During the strongest cold snaps, fishes and sea turtles can become and lethargic or fatally cold-stunned. The Texas Marine Cold Water Response Collaborative (TCRC) alerts organizations such as the Gulf Intracoastal Canal Association (GICA) to interrupt navigation during the recommended period needed for conservation groups to rescue sea life from these cold weather events. These predictive alerts reporting the onset and duration of cold stunning events are based on AI models developed by CBI in the late 2000’s, presently maintained and improved upon by CBI AI2ES members. The CBI AI2ES team continues work with collaborators to study how these AI predictions are understood and used by stakeholders.
Sea Turtle Conservation Model Updates:
- The AI operational prediction model (shallow neural net) was extended to 120 hours lead time facilitating the decision process ahead of cold stunnings.
- The team provided predictions and guidance for the determination and adjustment of the start and stop of navigation interruptions during a February 2022 cold stunning event.
- Continued preparation of the stakeholder engagement including tentative agreement from stakeholders such as the Texas Marine Cold-water Response Collaborative and Texas Parks and Wildlife to participate.
(reported by the National Science Foundation, 2022)
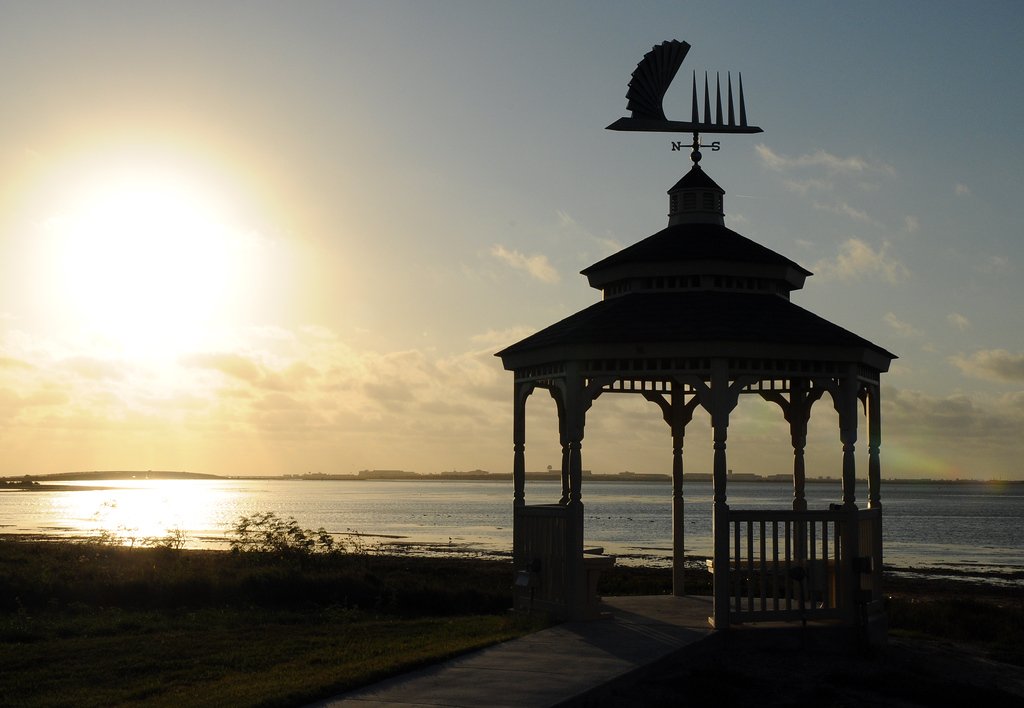
Coastal Fog Predictions (FogNet)
Coastal fog is a safety concern for transportation, particularly in a coastal city with frequent ship and plane travel. CBI AI2ES team has developed a sophisticated deep learning model, known as FogNet, which combines satellite sea surface temperatures and numerical model outputs to predict the onset of fog with 9 to 24 hours advance warning. The fog models are presently in their research stages, but AI2ES collaborators including CBI, the National Center for Atmospheric Research (NCAR), and The Weather Company/IBM, are working together to create operational models that are high performing, cost-effective, and meet most of the expectations of future users.
FogNet - Coastal Fog Prediction Updates:
- Paper “Importance of 3D convolution and physics on a deep learning coastal fog model” applying XAI to FogNet (Kamangir et al.) accepted in Environmental Modeling and Software.
- FogNet results including XAI are used as part of a collaboration with risk communication.
- The collaboration with risk communication has led to shifting FogNet to probabilistic outputs.
- As part of a collaboration with IBM a VAE alternative to FogNet is being explored (would be easier to implement operationally).
(reported by the National Science Foundation, 2022)
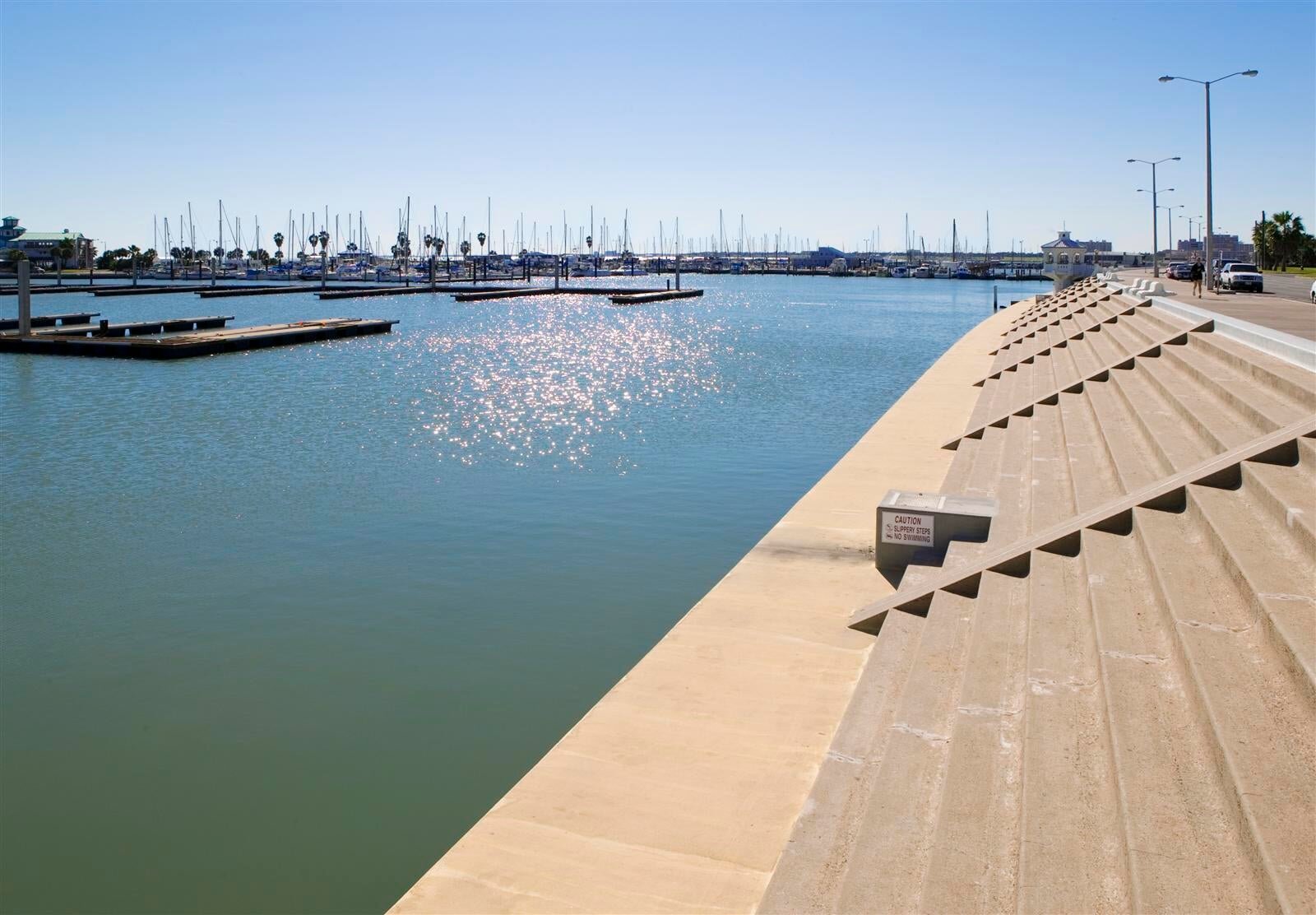
Semi-Automating Research-to-Operation
of AI Models with Python (Semaphore)
Having a simplified process to bring research models to operation (R2O) is highly beneficial but a challenge given the constant changes in both technologies and modeling approaches. Operationalizing the models helps deploy and communicate predictions to stakeholders and the public generating exposure and feedback. A rapid transition to at least the prototype or local stage is critical to obtain this feedback and be able to more realistically co-produce operational models with future users. Semaphore is a python program that semi-automates the operationalizing AI models, at present models that can be saved as .H5 files. Semaphore uses an agile design, specific data objects, an easily expandable data ingestion module, and a database to support a wide variety of data series. It uses a combination of .H5 files and Semaphore-specific data specification files to be able to interact with AI models regardless of data or design. Data is standardized to a unique set of keys that allow Semaphore to understand any data series. This helps series information to be easily communicated, saved, and queried. Semaphore will ease the burden of operationalizing a model from researchers, allowing the process to be quicker.
Semaphore has:
- Been presented at Baltimore’s 104th annual American Meteorological Society convention on February 1st, 2024.